Enhancing ABA with Scatterplot Insights
Unlock the power of scatterplots in ABA! Gain valuable insights and enhance your path to success.
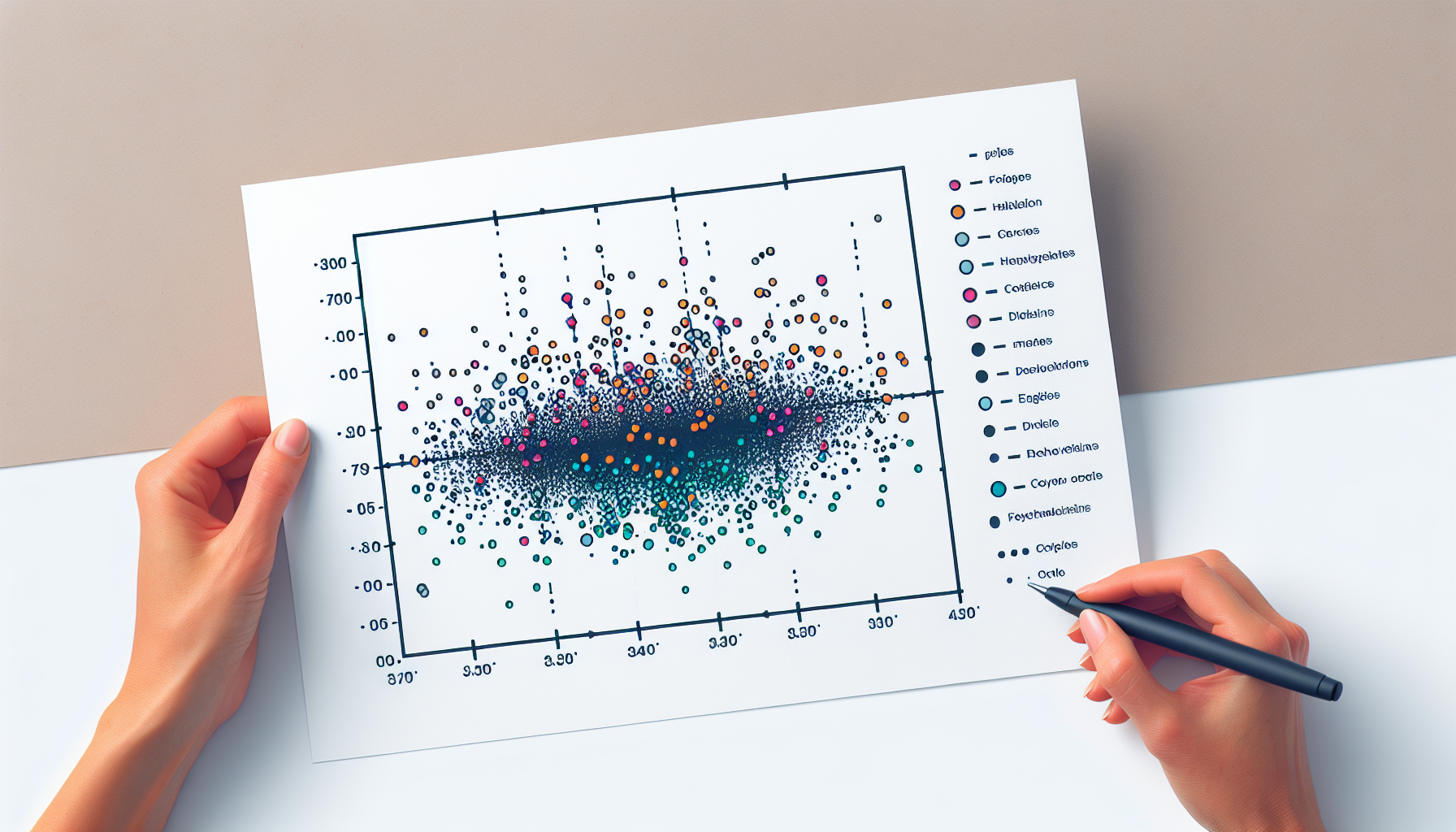
Understanding Scatterplots in ABA
A scatterplot is a powerful tool utilized in Applied Behavior Analysis (ABA) to analyze and interpret data related to behavioral patterns. It provides valuable insights into the relationship between different variables, helping ABA clinics and practitioners make informed decisions and develop effective intervention strategies. In this section, we will explore the importance of scatterplots and their applications in ABA clinics.
Importance of Scatterplots
Scatterplots play a crucial role in ABA by helping behavioral technicians identify and understand different behavioral patterns of concern. They provide critical information about when certain behaviors happen and the setting in which they take place. This information enables ABA centers to pinpoint appropriate behaviors or activities during the observation of client conduct, ultimately leading to more effective interventions.
The biggest advantage of scatterplots in ABA is that they display the connection between a client's behavior and their progress over time. This makes patterns in behavior more observable and easier to assess, even nonlinear patterns resulting from routine changes during therapy. By analyzing scatterplots, ABA professionals can identify trends, track progress, and make data-driven decisions to enhance treatment outcomes [1].
Applications in ABA Clinics
In an ABA clinic, scatterplots are used to help teams of therapists identify circumstances concerning the routines of patients and clients. The data collected through scatterplots is aimed at boosting or mitigating certain behaviors of interest or problematic behaviors. By analyzing the scatterplot, ABA practitioners can identify potential triggers, develop intervention strategies, and monitor the effectiveness of their interventions [1].
Scatterplots are not only utilized in ABA clinics but also by special needs teachers and parents. They can be particularly helpful in treating children with autism who exhibit behavioral problems ranging from small to severe, such as tantrums. By gathering data and analyzing scatterplots, these professionals can gain valuable insights into the behaviors of their students or children, enabling them to provide targeted support and interventions [1].
By leveraging the power of scatterplots, ABA professionals can gain a deeper understanding of behavioral patterns, track progress, and make informed decisions to enhance the effectiveness of interventions. The application of scatterplots in ABA clinics and related settings offers valuable insights that contribute to the development of individualized treatment plans and the overall well-being of individuals receiving ABA therapy.
Data Collection with Scatterplots
In Applied Behavior Analysis (ABA), scatterplot analysis is a valuable data collection method that helps professionals draw correlations between the time of day and behavior. By utilizing scatterplots, ABA practitioners can gain insights into the relationship between variables and identify patterns and triggers related to specific behaviors.
Data Analysis and Correlations
Scatterplot analysis involves graphing data points on a scatterplot to visually represent the relationship between two variables. In the context of ABA, the x-axis typically represents time or sessions, while the y-axis represents the frequency, duration, or intensity of a target behavior. By plotting behavior data over time, professionals can observe trends, patterns, and correlations that may not be apparent through other data collection methods.
One of the primary purposes of data analysis using scatterplots in ABA is to examine correlations between the time of day and specific behaviors. By segmenting time into small blocks and marking a symbol in each block each time a behavior occurs, professionals can identify time blocks with more symbols, indicating a higher frequency of the behavior. This process helps pinpoint potential triggers and allows for targeted intervention strategies [2].
Identifying Behavioral Patterns
Scatterplot analysis in ABA is particularly useful for identifying behavioral patterns. By visually analyzing the scatterplot, professionals can identify trends and patterns in the data. For example, they may observe that a certain behavior occurs more frequently during specific time periods or in certain environmental conditions. This information can help guide intervention strategies and inform behavior management plans.
Moreover, scatterplots allow professionals to track progress and assess the effectiveness of interventions over time. By comparing scatterplots before and after implementing interventions, practitioners can determine if the intervention has resulted in positive changes in behavior. If progress is not evident, adjustments or modifications to the intervention plan can be made to better meet the individual's needs.
Overall, the use of scatterplots for data collection in ABA enables professionals to analyze correlations, identify triggers, and track progress. This visual representation of data helps practitioners make informed decisions about intervention strategies and tailor treatment plans to effectively address target behaviors.
Benefits of Scatterplot Analysis
Scatterplot analysis plays a vital role in Applied Behavior Analysis (ABA) by helping professionals track progress, evaluate the effectiveness of interventions, and make data-informed decisions. Let's explore two key benefits of utilizing scatterplot analysis in ABA: progress tracking and intervention effectiveness.
Progress Tracking
Scatterplots provide a visual representation of data points, allowing for a comprehensive view of progress over time. By plotting data on a scatterplot, professionals can track changes in behavior, skill acquisition, or other targeted variables. This visual representation helps identify trends, patterns, and fluctuations in the data [4].
Tracking progress through scatterplot analysis enables professionals and parents to set realistic goals, monitor skill development, and make informed decisions about intervention strategies. By regularly updating the scatterplot, it becomes easier to visualize progress and identify areas that may require additional support or intervention adjustments.
Intervention Effectiveness
One of the primary purposes of scatterplot analysis in ABA is to evaluate the effectiveness of interventions. By plotting data points before and after implementing an intervention, professionals can assess its impact on the targeted behavior or skill. This analysis helps determine if adjustments or modifications to the intervention plan are necessary.
Scatterplots allow professionals to compare data points over time, helping them determine if the intervention is producing the desired changes. If the scatterplot shows a positive trend or a reduction in the targeted behavior, it indicates that the intervention is effective. On the other hand, if there is no significant change or a negative trend, it may be necessary to reevaluate the intervention approach and make appropriate modifications [5].
Analyzing the scatterplot data allows for data-driven decision-making, leading to more effective intervention strategies. It provides insights into which interventions are yielding positive results and helps professionals identify the most effective techniques for each individual.
By utilizing scatterplot analysis for progress tracking and evaluating intervention effectiveness, professionals in the field of ABA can make informed decisions, set achievable goals, and celebrate the positive impact of interventions. With a data-driven approach, professionals and parents can work together to support individuals in reaching their full potential.
Practical Use of Scatterplots
Scatterplots are a valuable tool in Applied Behavior Analysis (ABA) for understanding relationships between variables and identifying patterns in data. In this section, we will explore two practical applications of scatterplots: time-trigger correlations and outlier identification.
Time-Trigger Correlations
One practical use of scatterplots in ABA is to analyze time-trigger correlations. This method helps professionals draw connections between the time of day and specific behaviors on a broader scale. For example, scatterplot analysis can assist in determining if a student exhibits more frequent instances of hitting other children during art class. By segmenting time into small blocks and marking symbols for each occurrence of the behavior, professionals can identify time blocks with higher frequencies of the behavior, helping pinpoint potential triggers.
A scatterplot illustrating time-trigger correlations can provide valuable insights into the temporal patterns of behaviors. By plotting the time of day on the horizontal axis and the frequency or intensity of the behavior on the vertical axis, professionals can visually identify any relationships or patterns that may exist. This information can guide intervention strategies and help in developing behavior support plans.
Outlier Identification
Another practical use of scatterplots in ABA is to identify outliers within the data. Outliers are data points that deviate significantly from the general pattern or trend observed in the scatterplot. These points may have a notable impact on the analysis and provide valuable information about unique or extreme occurrences in the behavior data.
By examining a scatterplot, professionals can visually identify data points that are distant from the main cluster of points. Outliers can represent important events or circumstances that affect behavior and may require further investigation or targeted intervention strategies. The ability to identify these anomalies allows for a more comprehensive understanding of the data and enhances the accuracy of analysis.
In summary, scatterplots are a powerful tool in ABA for practical data analysis. They enable professionals to identify time-trigger correlations, helping to pinpoint potential triggers for specific behaviors. Additionally, scatterplots assist in identifying outliers, providing valuable insights into unique occurrences within the data. By utilizing scatterplots effectively, professionals can enhance their understanding of behavior patterns and make informed decisions regarding intervention strategies.
Interpretation of Scatterplots
Scatterplots are a powerful tool in Applied Behavior Analysis (ABA) that allow professionals to easily visualize and analyze data. In the interpretation of scatterplots, two key aspects to consider are correlation analysis and data pattern recognition.
Correlation Analysis
Correlation analysis is a fundamental aspect of interpreting scatterplots. By examining the distribution of data points on a scatterplot, professionals can gain insights into how changes in one variable may be associated with changes in another variable. This analysis helps identify the strength and direction of the relationship between the variables.
Positive correlation indicates that as one variable increases, the other variable also tends to increase. In contrast, negative correlation suggests that as one variable increases, the other variable tends to decrease. The strength of the correlation is determined by how closely the data points align along a trendline or cluster together.
It's important to note that correlation does not imply causation. While a strong correlation may exist between two variables, additional research and analysis are necessary to establish a causal relationship.
Data Pattern Recognition
Data pattern recognition is another crucial aspect of interpreting scatterplots. Scatterplots help ABA professionals identify trends and patterns by visualizing the data points collected. By observing the overall distribution of data points, professionals can identify common patterns or anomalies.
Patterns can include linear relationships, where the data points roughly follow a straight line, or nonlinear relationships, where the data points form a curved or irregular pattern. These patterns can provide valuable insights into the behavior being analyzed and guide decision-making in ABA practices.
Additionally, scatterplots can identify outliers and anomalies within the data. Outliers are data points that are distant from the other data points and may have a notable impact on the analysis. These outliers may represent unique cases or measurement errors, and their identification is important for accurate analysis and interpretation.
By utilizing correlation analysis and data pattern recognition, ABA practitioners can gain a deeper understanding of the data collected through scatterplots. This understanding enables data-informed decision-making, progress tracking, and evaluation of intervention effectiveness in ABA practices.
Best Practices for Scatterplot Use
To make the most of scatterplots in Applied Behavior Analysis (ABA), it is important to follow best practices for data visualization and interpretation. By employing these practices, you can effectively analyze and communicate your data while avoiding misinterpretation.
Data Visualization Techniques
When creating scatterplots, it is essential to employ effective data visualization techniques. Here are some best practices to consider:
- Plotting Data Points: Ensure that each data point is accurately represented on the scatterplot. Use distinct symbols or colors to differentiate between different data sets or conditions, if applicable.
- Axis Labels and Units: Clearly label the x-axis and y-axis with appropriate titles and units, providing a clear understanding of what each axis represents. This enables viewers to interpret the data accurately.
- Scaling and Range: Choose appropriate scales for each axis to avoid distorting the data. Ensure that the range of the axes includes all relevant data points. This allows for a comprehensive understanding of the data distribution.
- Title and Captions: Include a descriptive title for the scatterplot that reflects the purpose of the analysis. Additionally, consider adding captions or annotations to highlight important observations or trends within the data.
Avoiding Misinterpretation
Though scatterplots are powerful tools, misinterpretation can occur if caution is not exercised. Here are some best practices to avoid misinterpretation:
- Correlation vs. Causation: Recognize that correlation does not imply causation. Be cautious when inferring cause-and-effect relationships based solely on scatterplot data. Additional analysis and evidence are often required to establish causal links.
- Overplotting: Overplotting occurs when multiple data points overlap, making it difficult to distinguish individual data points. To avoid this, consider using transparency, smaller data point sizes, or density plots to display density within crowded areas of the scatterplot.
- Outliers: Identify and handle outliers appropriately. Outliers can significantly impact the overall trend and interpretation of the data. Consider investigating the reasons behind outliers and determining whether they should be excluded or further analyzed.
By following these best practices, you can effectively utilize scatterplots in ABA to visualize and interpret your data. Remember that clear and accurate visualization is key to ensuring that your scatterplots provide valuable insights for making informed decisions, tracking progress, and collaborating effectively.
References
- https://www.crossrivertherapy.com/aba-therapists/scatterplot
- https://accupointmed.com/measuring-behavior-seven-aba-data-collection-methods/
- https://www.supportivecareaba.com/aba-therapy/scatterplot-in-aba
- https://www.abataba.com/blog/scatterplot-in-aba
- https://www.bridgecareaba.com/blog/scatterplot-in-aba/